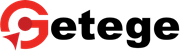
Statistics plays a pivotal role in data science by providing the necessary tools and techniques to analyze, interpret, and draw conclusions from data. Whether it's understanding customer behavior, optimizing business processes, or predicting future trends, statistics forms the foundation of data-driven decision-making.
Descriptive statistics means Descriptive statistics encompass the process of summarizing and elucidating the features and attributes inherent within a dataset. encompass the process of summarizing and elucidating the features and attributes inherent within a dataset.Measures of central tendency, such as mean, median, and mode, provide insights into the typical value of a variable, while measures of dispersion, like variance and standard deviation, quantify the spread of data. Visualization techniques, including histograms, box plots, and scatter plots, offer intuitive ways to explore and communicate data patterns.
Inferential statistics enable data scientists to make inferences or predictions about a population based on sample data. Through hypothesis testing, confidence intervals, and regression analysis, researchers can assess the significance of relationships, identify patterns, and make probabilistic statements about future outcomes.
Probability theory serves as the mathematical framework for quantifying uncertainty and randomness. Understanding the basics of probability, different probability distributions, and concepts like Bayes' theorem is essential for modeling uncertain events and making probabilistic predictions.
The applications of statistics in data science are diverse and wide-ranging. From predictive modeling and A/B testing to anomaly detection and clustering, statistical methods are instrumental in extracting actionable insights and driving business outcomes.
While statistics empowers data scientists to extract valuable insights, it also poses certain challenges and limitations. Issues such as data quality, overfitting, and misinterpretation of results can hinder the effectiveness of statistical analyses and lead to erroneous conclusions.
To mitigate these challenges, adopting best practices is crucial. This includes rigorous data preprocessing to ensure data integrity, selecting appropriate statistical techniques based on the nature of the problem, and validating results through robust methodologies.
Looking ahead, the integration of machine learning with traditional statistical methods, advancements in big data analytics, and heightened awareness of ethical considerations are shaping the future landscape of statistics in data science.
In conclusion, statistics serves as a cornerstone of data science, enabling organizations to extract actionable insights, make informed decisions, and drive innovation. By embracing statistical methods, businesses can unlock the full potential of their data and stay ahead in an increasingly competitive market.
Statistics provides the necessary tools and techniques to analyze, interpret, and draw conclusions from data in data science.
Common applications include predictive modeling, A/B testing, anomaly detection, and clustering.
Challenges include data quality issues, overfitting, and misinterpretation of results.
By harnessing statistical methods, businesses can extract meaningful insights from data, make informed decisions, and drive innovation.
Future trends include the integration of machine learning, advancements in big data analytics, and heightened awareness of ethical considerations.